What is the Value of Human-Level AI to Education?
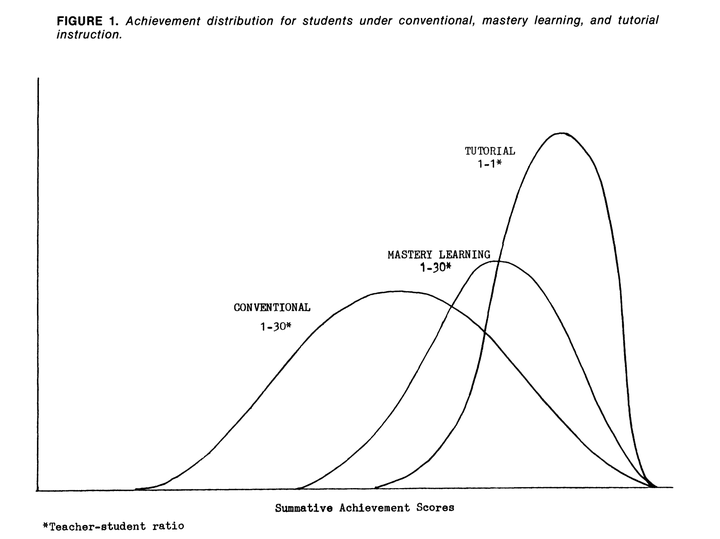
This post is co-written with Dean Foster and Sham Kakade
Growing up in Calcutta, I was probably 8 years old when I first noticed the stark poverty around me. I can’t remember anything before or since that’s moved me more than the lost life I saw in large parts of the developing world. It’s a big part of why Poor Economics became one of my favorite books within the few hours it took me to finish the first 3 chapters. I had finally seen someone approach the problem with humility, data, and a great deal of empathy. Since that day, I’ve obsessively tracked a lot of the literature that’s come out of the field of Developmental Economics.
Dean’s father worked as a Professor in Agricultural Economics, and so he spent most of his childhood seeing a lot of the same poverty I saw. I could hardly believe an American academic who spent his life working in CS/Stat/AI felt the same empathy I did. So, we spend a great deal chatting about development and how we can help.
Sham’s belief that humanist goals generally focus on where we can make the most impact, and the mandate at the Kempener Institute to apply AI technologies to the benefit of humanity had him onboard almost immediately.
The three of us being big believers in AI, and in the general intelligence of language models, realized that this might be the first time a technology could be cheaply deployed to educate a large number of children at a very low cost. We thought that this might make it a perfect candidate for education in the developing world, so when Jacob invited me to speak at the Human-Level AI Workshop in Boston1 - I realized I could use the talk to flesh out my thoughts on why we think that this might be the start of a series of game changing experiments.
So this post will go over my talk in a bit more detail. It doesn’t matter if you believe the current language models are intelligent or not, I hope I can convince you that they might still be very valuable for this problem; and that this problem matters.
Social Returns to Education
One of the questions economists deeply worry about is what they call the “social returns” to a policy. The idea being that if we’re going to invest heavily in some policy (say educating people) how much does society hope to benefit. These estimates are not meant to be precise to the 4th significant digit, but rather within an order of magnitude.
One of the ways that economists might measure the returns on education is to ask how much an additional year of education increases wages. So, they might write an equation that looks something like:
$$ \text{ln}(w) = f(s, x) = \text{ln}(w_0) + \rho S + \beta_1 x + \beta_2 x^2 $$
where $w$ represents earnings ($w_0$ being the earnings of a person with no education), $S$ being the number of years of schooling and $x$ representing the years of work (labor market experience). Under this model, the parameter $\rho$ would represent the marginal value (in wage growth) of an additional year of education.
This equation, called the Mincer Equation, has been estimated in many different ways many different times and the numbers typically range anywhere from a 5-10%
There are (somewhere close to) 2.2 billion students in the world today who are supposed to be in primary or secondary school, with an estimate of 1.4 billion of those children living in low and middle income countries. If we assume that their wages will grow according to these estimates, that would make the value to world GDP about ~$5TN (see Appendix A for a more detailed calculation). That’s a really large number! Now, of course you could argue that the Mincer equation only estimates an average value, and that the price (wage improvement) of education will go down as more people become educated. That’s fair enough. But there is a large body of evidence that education might be a goal in itself. People who are educated are more likely to believe in Democracy, make better health decisions, trust others, and report satisfaction with their jobs (though the evidence on that is a bit mixed).
None of these stories is known to be causal on its own, but there is a sense in which they all add evidence (the glass of wine causality model as Dean likes to call it) to the claim that education improves quality of life, and has a lot of spillover effects.
 for educated and uneducated men and women (top) and the [median earnings by educational attainment](https://www.bls.gov/careeroutlook/2018/data-on-display/education-pays.htm) (bottom). On the right, we have a correlation between [child mortality](https://ourworldindata.org/global-education#the-consequences-of-education) and the average number of schooling years for women in a country (top) and [measures of democracy](https://ourworldindata.org/global-education#the-consequences-of-education) (ranging from 0 to 1 - where 1 is the most democratic) vs past average years of schooling (bottom).](/post/llms_education/macro_hu49d3a5fb660e3369e98992253f6a8c13_417659_908458a87a7394bf6842de2ef28efe9c.png)
What are the problems facing education today?
Since reading Poor Economics, I’ve always thought that the problems in education could be categorized as - 1) Structural and 2) Economic.
I think of Structural Issues as those that arise as an epiphenomenon of another problem. Teacher Absenteeism is a rampant problem in the developing world - nearly 20-25% of the time a teacher is supposed to be standing in front of a classroom, there isn’t one. And the evidence seems to say that the situation isn’t improving.
Now, a classic argument in economics is that when there is demand for a good - people will pay for it, and the problem will get solved. That’s a bit reductive, but it is the spirit of what Banerjee and Duflo call the “Demand-Wallah” arguments. The critical issue with this reasoning for education is that it’s the parent who pays, and (mostly) the child who gains. So when parents suffer from a miscalibration of expectations with regard to education, it’s the kids who have to suffer the effects of the “winner take-all” policies.
On the flip side, there are deep economic issues that slow down progress here. Educating a child isn’t cheap, even in the developing world. The World Bank estimates a lower bound of about US $200/child/year, putting the cost of educating the 600 million children out of secondary school at about $120 billion/year. And that isn’t even the major cost! Every hour a child spends in school is an hour they aren’t working, which is a real “opportunity cost” when money is (very very) tight.
 estimate for percent attendance based on school level. A definition for [LDC can be found here](https://www.un.org/ohrlls/content/list-ldcs).](/post/llms_education/attendance_huf48992c8fc3e5f3a707a2c73b5b24d01_73020_7aa263d11ee8b3a536db02297df2f069.png)
The figure above shows observational evidence in support of this - kids are more likely to be out of secondary or upper secondary school (in the world and its least developed countries). One of the factors that might cause this is that each hour of their time is more valuable as they get older, which means education needs to return more. There is evidence that the bottom third of a class in an LDC gets close to 0 value-add from an additional year of education. This would support the idea that the real issue isn’t infrastructure or availability, but simply the fact that a lot of people in an LDC don’t get much out of their education and so there isn’t much demand for it.
So, what works and what doesn’t?
Banerjee and Duflo (as well as Muralidharan) go over a lot of the experiments that have been run to try and improve learning outcomes in developing countries. There are two broad classes of solutions - “Demand Side” and “Supply Side”.
Let’s start with the Demand Side, where the picture is (relatively) rosy. The key idea of demand-side interventions is that we may be able to take some action to spur demand. That could be increasing the returns to education by providing more jobs - something that happened when India saw a massive surge in call center jobs for young women, or when the Green Revolution accelerated farming yields through new technology.
There are a number of demand side interventions which have shown promising, though prohibitively expensive, outcomes. Student level incentives, information-sharing, as well as cash transfers (for which there are numerous experiments) have all demonstrated significant improvements in learning outcomes. The problem with a lot of these interventions is that they’re prohibitively costly. This might be part of why so many different supply side interventions have been tried.
The Supply Side picture is a lot more dour. I once saw a talk by Karthik Muralidharan who said something along the lines of (I’m paraphrasing) “the expected benefit for providing resources can be summarized as 0.” I kind of agree! A ton of trials which try to provide resources to schools seem to have zero to little impact. But, there is one thing that does decidedly work. And I think that one supply side intervention is what LLMs and Human Level AI (HLAI) can bring to the table.
Tutoring
In 1984, Bloom wrote an influential paper where he showed that 11 additional periods of instruction (in a 1:1 setting - or as he called it “Tutorial Instruction”) over a 3 week block of time produced a nearly 2 standard deviation improvement in test scores. The effect was so large that the problem of finding group instruction techniques that are as effective as one-to-one tutoring has earned the moniker of Bloom’s 2 sigma problem. This effect has since been replicated (with varying but almost uniformly positive results) and the consensus is that tutoring really does work.
So, why is that? Is it simply additional instruction time? Certainly that helps - but kids are better off in during-school sessions with tutors (even when they aren’t trained) then in after-school sessions2, so something else might be going on. Some of the hypotheses that have been put forward include:
-
Additional Instruction Time: One of the primary mechanisms by which Tutoring might help students is by simply providing them with more class time.
-
Personalization: Another plausible mechanism by which tutoring might see such large effect sizes is if tutors are tailoring their instruction to each student. In a large classroom, a teacher is forced to teach to the mean/median student - where as in a 1:1 setting the tutor can identify the errors a student is making and guide their instruction according to those. Personalization is one of the things I think Human-Level AI can seriously help with, even if it isn’t as good as human teachers from the get-go.
-
Rapid Feedback: Tutors can likely provide feedback on errors at a much higher rate than a teacher speaking to many students - which might allow students to remain more on task (focused/engaged) than in classical group instruction settings.
-
Mentor Relationship: Another plausible mechanism by which tutoring helps is if students develop a personal relationship with their mentor, allowing the mentor to exert more patience and personalization towards the student then in a group setting.
I think Human Level AI could help with a lot of these3, but especially with personalization and feedback. There’s growing evidence that personalization might be one of the largest things that tutoring brings to the table (beyond more instruction time).
Personalization
Probably the mechanism I’m most excited by is called Customization of Learning (or Personalization). The idea being that a tutor might vary the nature of their instruction based on a student’s needs. This is certainly a plausible model, since in a large classroom - if there is variability in student learning rates, the bottom third of a class might be the bottleneck for average class-time productivity. While no experiment I could find has varied the degree of personalization (which would help us understand the effects of the personalization itself) there is a growing body of evidence that suggests that the “degree” of personalization may be an important covariate to the improvement in learning outcomes.
One way of thinking about tutoring is to say that it decreases the teacher-student ratio all the way to 1 (it’s usually around ~30 in America, and much more in the developing world). But we could ask what happens when we decrease the teacher-student ratio at all. There are a few experiments that try to do this, so it’s worth going through them.
The first experiment that convinced me is usually called the Balsakhi experiments that Banerjee et. al (2007) ran in India. The idea was that children who had reached the 3rd or 4th grade without basic skills would be pulled out of regular school and paired with a community member (with little training), a balsakhi (child-friend), who would provide them with remedial help. The RCT found large effects on test scores - partially indicating that while trained tutors might provide the largest benefits, tutoring time in general can have positive effects.
The other experiment I read about was the one that Duflo et. al (2011) ran in Kenya - which was an implementation of what they called “Teaching at the Right Level”. What they found was that when classrooms were split (this was done in a randomized way) according to learning level, students across the board benefitted from tracking.
While Muralidharan et al (2018) weren’t able to experimentally vary the degree of personalization - they argue that it was one of the major drivers for the benefits that the Mindspark program showed.
While I’ve yet to find an RCT where the degree of personalization is itself varied - the evidence is growing that this might be one of the major mechanisms through which tutoring provides benefits to students.
Human Level AI
This is where HLAI comes in. Marc Andreessen recently put tutoring atop his list of the benefits from AI, saying “Every child will have an AI tutor that is infinitely patient, infinitely compassionate, infinitely knowledgeable, infinitely helpful”. I’m certainly sympathetic with that, but I’d add one (important) caveat - at a low cost. I think the great promise of HLAI comes from the fact that we might finally have a solution that is able to provide a lot of the things we’d like - without being economically untenable.
So my mantra for how HLAI will impact education in the developing world is cost, cost, cost. Whether the cost reduction comes from the fact that it can be done through any smart-phone (a reduction in travel cost), or flexibility to the students labor schedule (a reduction in opportunity cost), or simply because training an AI model in every subject4 is much cheaper than training (or re-training) a teacher (a reduction in labor cost) - remains to be seen.
Current Efforts
 (left) and Plastic Labs' [tutor-gpt](https://github.com/plastic-labs/tutor-gpt) (right)](/post/llms_education/tutors_hu34f368ebcc41c5f397c0ee1f11f14fda_346597_273d3ff23e75d4a53d0e2b14821cf3fd.png)
I’ve been looking into what efforts there are today - and I found a few major ones, so people are definitely thinking about this. tutor-gpt is a LangChain App that seems inspired by theory-of-mind experiments. Khan Academy has an LLM chatbot that might be using techniques like Chain of Thought prompting, citations, and maybe RLHF for engagement to create an experience that seems pretty good! Teachers are already putting it to the test - and the anecdotal evidence seems mixed, but I don’t think that’s too representative.
I think of the major issues that Technology Assisted Learning (also called Computer Assisted Learning/CAL) has had is that a lot of the experiments have been run in the developed world/the United States. I mean, if we just look at Table C1 in the Appendix of Muralidharan et al’s MindSpark paper, row after row for the experiments run in the US end with “no-effect”. The problem with that is Control (in the RCT sense) is a really high bar! Its a well-trained US teacher. The “external validity” of this experiment isn’t necessarily relevant to the developing world - where Control might not meet this high bar. This might be why Banerjee et al, Yang et al, and Lai et al saw much better effects than what the US experiments would have predicted.
What issues might we run into?
But that doesn’t mean every problem is solved. There are still some issues that I don’t think HLAI can help with. Some of them might be:
- Fixed Cost: A lot of this post assumes that every child who needs to be educated has access to a device that can support an AI model. This might not necessarily be the case, and the fixed cost of getting each child such a device might be prohibitive.
- Technology isn’t good enough/reliable enough yet: The first versions of the AI Tutors might not be as good as humans, or have the reliability of human teachers. They may not be engaging enough for students to spend a lot of time with. I think these are definitely very valid criticisms, but I think it’s not all or nothing.
When Duflo (2001) did her famous natural experiment on the INPRES Program she found that the returns to education were basically linear in the number of years (of education). The Barro-Lee (2016) datasets find the same effects in many different countries. I think the key take away from these graphs is that education isn’t all or nothing, that every little bit helps and that’s why I’m optimistic even though I agree with the criticisms above about reliability and engagement.
 (left) and [Barro-Lee](http://pamjakiela.com/arec345lecture16_handout.pdf) (right)](/post/llms_education/returns_hud54fedd12029c009cb3930c326d40c38_308754_52210c60d80283ca98ad0c4dae991154.png)
- Low Resource Languages: A lot of the current LLMs are trained on languages for which there is a lot of textual data (English, French etc.). But a lot of the developing world speaks languages that don’t have as much information on the web. So we may need to improve our models on these Low Resource Languages to see large impacts from them.
- Bias Issues: One of the major open research questions I can think about is how we can handle the problems present in the datasets we might use to train these AI tutors.
When Claude Steele and his co-authors did their famous experiments, they found that even simple interventions - like telling women there were no gender differences in a test - removed a lot of the underperformance that was associated with women, indicating that the “stereotype threat” is “present in our data” in some way.
This is relevant for the developing world too, since similar experiments have found that randomly exposing the name of a subject changes the performance on a task - indicating that if we use data “labeled” by teachers, our models might inherit the bias present in that data. How we handle this issue is both important, and an open research question.
What I want to work on
To make progress, I think there are a few things we need to accomplish. This is my (not comprehensive at all) list of things I’m either thinking of or currently working on. If you think I’ve missed something, I’m very excited to hear about it!
- Benchmarks: A lot of AI (and LLMs in particular) has a whole suite of benchmarks that have served as reliable “surrogate/proxy endpoints” for performance on downstream tasks. Models that won the ImageNet challenge soon became the state of the art for a variety of business specific use cases that don’t seem that related to ImageNet. Similarly, I think we need benchmarks that might reliably predict the improvements in learning outcomes we hope to see when we start large-scale RCTs of these AI tutors in the developing world! What these benchmarks are, and how we’re going to validate them, is one of the most important stepping stones I can see.
- Methodological Improvements: It’s not clear whether prompt engineering itself can allow LLMs (or other HLAI models) to provide personalization and rapid feedback to each student individually. We may need RLHF or other techniques to increase the personalization and improve the feedback from LLM/HLAI models, or we may need to develop new science - which I find incredibly exciting!
- Evidence: Banerjee and Duflo certainly only increased my belief in the value of experiments, and I think RCTs should be the “gold-standard” by which we judge the efficacy of our AI tutors (there is already effort in this direction). But, we should probably also run some “Piaget” style experiments and observe children interacting with these tutors. The idea would be that these (much easier/cheaper) experiments might quickly identify major holes in our models or our beliefs of their efficacy!
Wrapping up
Thanks for struggling through this. We (DDS)5 genuinely believe that education might provide some of the largest social value from HLAI. Even if the first few versions of the software aren’t “quite there yet” - I do think every little bit helps, and that we can provide a lot of value to people through this technology. We’re very excited to collaborate with people on research, experiments, deployments, or just ideas - so please, reach out if you’re working on this and would like some help!
Appendix A: Where does our 5 trillion come from?
It’s not easy to come up with a single number that quantifies the value we think HLAI will bring, but we can try a few educated guesses. In this section, we’ll consider a pessimistic scenario - and try to show that this would still be a hugely positive investment.
For every child, we will assume that the student will work with the tutor for 10 years. This isn’t a huge assumption, especially if we can get both student and parent to engage with the tutor and see the value the student is getting. Let’s also assume that students will continue their growth over the years. While the bottom third of a class might not be getting much value from a year of education today - the core assumption which we hope we have justified in this article is that a tutor will change this.
So if we believe the estimates of ~7% wage growth (see also Harmon et al (2000)) in a year, compounding would give us a factor of two growth ($2 \approx 1.07^{10}$.) The world poor earn about $ 2/day. If we can get the world poor to engage with the tutor, this would double their income to USD $4 /day. That’s a 100% increase, and there is evidence that this constitutes significant changes in quality of life. A typical person works about ~2000 hours a year. If we double the income of the 1.4 billion people in this group 20 years out that would add an extra 700 billion dollars a year in World Income, the Net Present Value (NPV) of which equals 5 trillion dollars today6.
We need to compare this cost of setting this up. Each of the 1.4 billion people will require a smartphone, giving us a $140 billion cost for hardware. Assuming each person requires $100 per year for inference (again this is a pessimistic scenario) - the total cost per year would be $ 140 Billion. Adding this up over 10 years gives us a total cost (discounted) of about $1.4 trillion. That gives us a ~400% Return on Investment (ROI), something we can’t remember having seen in the modern world!
Likely the actual value delivered will be far larger, but we’ll save that discussion for another post7.
-
If you’re interested, my slides are here ↩︎
-
ok fine, maybe not the Mentor Relationship one ↩︎
-
The GPT-4 Technical Report claims that GPT-4 is already at the proficiency of an American High School student in many subjects. ↩︎
-
Dean, Dhruv, Sham ↩︎
-
1.4 billion * 250 days/year * $2/day * $\sum^{\infty}_{n=20} 0.95$, assuming a discount factor of about ~5% ↩︎
-
If you’re curious, feel free to shoot us an email and we’d be happy to share the details of our calculations! ↩︎