bqplot: An Interactive Plotting Library for the Jupyter Notebook
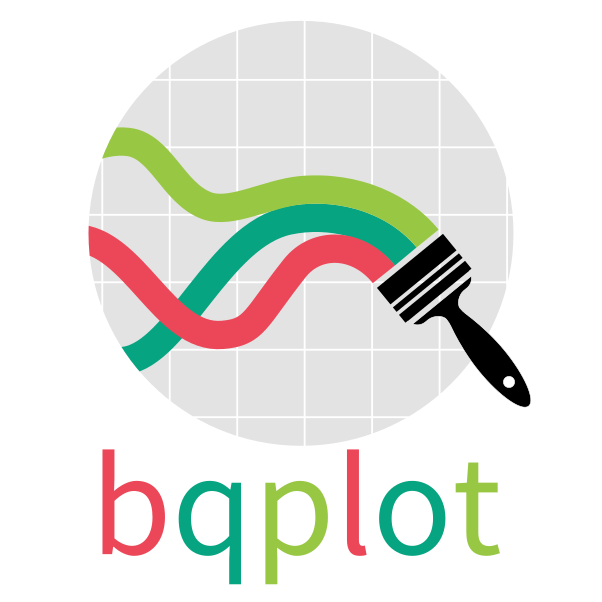
bqplot
Most of my open source work focuses on bqplot, an interactive visualization library for the Jupyter notebook. bqplot is based on the ipywidgets infrastructure, which I also used to work a great deal with.
One of the things (looking back at it) that I like best about bqplot is the object model API. It seems like a much more natural way to generate plots than some of the other Grammar of Graphics adaptations that I’ve seen. Partly I think because we were constantly shifting between application/widget development and library development - we represented users much better than other projects I’ve worked on.
For e.g. to generate a simple interactive scatter plot, first we import the requisite libraries:
import numpy as np
size = 100
np.random.seed(0)
x_data = np.arange(size)
y_data = np.cumsum(np.random.randn(size) * 100.0)
Then we import and declare the Scales, which are mappings from data
to pixels
.
from bqplot import LinearScale
# Let's create a scale for the x attribute, and a scale for the y attribute
x_sc = LinearScale()
y_sc = LinearScale()
Now we need to create the actual visualization (what we call the Mark). The Scatter
object takes in the x_data
, the y_data
and maps them to the passed scales like below:
from bqplot import Scatter
# Let's create a scale for the x attribute, and a scale for the y attribute
scatter_chart = Scatter(x=x_data, y=y_data, scales={"x": x_sc, "y": y_sc})
We also need to draw Axis
(which is the 1D visual representation of the Scale
). Notice that all that separates the x
-axis from the y
-axis is the orientation
parameter.
from bqplot import Axis
# Let's create a scale for the x attribute, and a scale for the y attribute
x_ax = Axis(label="X", scale=x_sc)
y_ax = Axis(label="Y", scale=y_sc, orientation="vertical")
Finally, we put it all together into a Figure, which we then display:
from bqplot import Figure
from IPython.display import display
fig = Figure(marks=[scatter_chart], title="A Figure", axes=[x_ax, y_ax])
display(fig)
The coolest part about bqplot is that this Figure
is a live-object (an ipywidget), and so is every component of it! So, we can change it!
scatter_chart.y = 2 * y_data
You should see something like this!
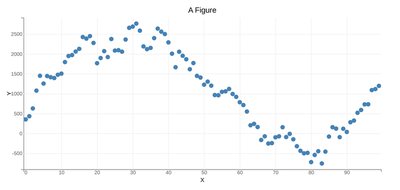
We can make the plot interactive by allowing it to be moved.
scatter_chart.enable_move = True
Go ahead, drag one of the points!
Now, here’s where the magic of widgets comes in. Let’s create a function foo
which will be called whenever the y
-data of the scatter chart is changed.
def foo(change):
print("This function is called whenever the y-data is changed!")
Finally, we tell scatter_chart
to call foo
whenever y
is changed. We do this by using observe
to register the callback.
scatter_chart.observe(foo, 'y')
If you move any of the scatter_chart
points, foo
should be called! That’s it! That’s all it takes to make an interactive plot in bqplot
.
bqplot Talks
Here’s a video of us speaking about it at Scipy 2017!
I gave a more extended talk at PyData Seattle
And at and PyData Ann Arbor
For some of our Deep Learning visualizations, have a look at my talks at PyGotham
And at PyData NYC
Licensing Information
This software was released under the Apache 2.0 license.